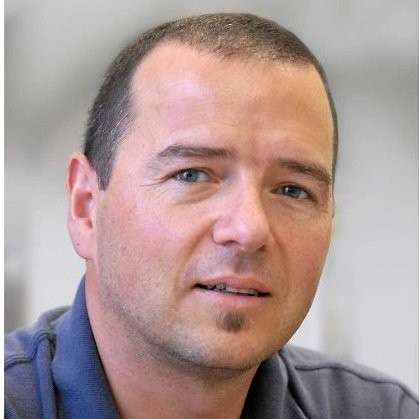
Podcast: Play in new window | Download () | Embed
Subscribe: Apple Podcasts | Spotify | Email | RSS | More
Demystify AI’s evolution, from Netflix recommendations to ChatGPT, exploring how neural networks learn & why even AI creators can’t fully explain how it works.
Summary
Claude AI used in this summary
🎙️ What the Heck is AI, Anyway? I sit down with tech expert Eric Pinaud to demystify artificial intelligence. Whether AI-curious or AI-anxious, this conversation breaks down complex concepts into digestible insights.
We explore:
• The evolution from Narrow AI (like Netflix recommendations) to today’s Broad AI (like ChatGPT)
• How AI learns through neural networks – similar to how we learn music or new skills
• Why even AI’s creators can’t fully explain how it works
• The environmental cost: ChatGPT’s daily operations cost $700k and use massive energy
Most importantly, we discuss how AI is becoming more accessible – anyone can ask questions and get answers, regardless of technical expertise. Though powerful, AI still needs human wisdom and judgment to be truly useful.
Click here to view the printable newsletter with images. More readable than a transcript, which can also be found below.
Contents
Please comment and ask questions:
- at the comment section at the bottom of the show notes
- on LinkedIn
- via email
- YouTube channel
- DM on Instagram, Twitter, TikTok to @healthhats
Production Team
- Kayla Nelson: Web and Social Media Coach, Dissemination, Help Desk
- Leon van Leeuwen: article-grade transcript editing
- Oscar van Leeuwen: video editing
- Julia Higgins: Digital marketing therapy
- Steve Heatherington: Help Desk and podcast production counseling
- Joey van Leeuwen, Drummer, Composer, and Arranger, provided the music for the intro, outro, proem, and reflection, including Moe’s Blues for Proem and Reflection and Bill Evan’s Time Remembered for on-mic clips.
Podcast episodes on YouTube from Podcast.
When Comic Books Predict Tech Better Than Scientists
Billions of Data Points Walk Into a Neural Bar…
Inspired by and Grateful to
John Marks, Amy Price, Dave deBronkart, Emily Hadley, James Cummings, Jeff Harrington, Jacob Sloane,
Links and references
Chester Gould introduced Dick Tracy’s 2-way wrist radio
The first notable AI development in the 1950s
Episode
Proem
Greetings. Welcome to the second episode in Health Hats’ occasional series, Artificial Intelligence Can Work for You. The first episode, AI: Neither Artificial nor Intelligent, demonstrated how I use artificial intelligence to make my multimedia DIY (Do It Yourself) sausage. A long-time reader, John, called to tell me he opens all my podcast notifications and emails yet never listens to anything smelling technical. But he opened this one, and for the first time, he had an inkling of what AI might be. Mission accomplished!
Let’s take another step and use our senses to picture this animal, Artificial Intelligence. How much can we recognize? What feels like sci-fi? What smells like BS? How much is inconceivable? Think of cell phone inventor Alan Gross, who conceived of the cell phone after comic strip creator, Chester Gould introduced Dick Tracy’s 2-way wrist radio in 1946? A path from nowhere in human consciousness to a figment of someone’s imagination in a cartoon (1946), then opening a mind to possibilities (1964), a concept and prototype (1973), and a product (1983). The Digital phone (1990), just thirty-five years ago. You know the rest
Intelligence
Let’s talk about intelligence for a minute. I frame human intelligence in several ways. One is that data can lead to information, sometimes to knowledge, and occasionally to wisdom. Another is how I think about health: Physical, Mental, and Spiritual Health. So, perhaps for Intelligence, Physical intelligence is clinical information about the body, Mental intelligence is feelings, and Spiritual intelligence is faith and ethics. So far, Wisdom, Feelings, Faith, and Ethics seem uniquely human.
Is AI Understandable?
Recently, I visited Eric Pinaud, my dear friend and go-to info tech translator. I needed help paddling through the AI soup. I can confidently discuss my AI experiments, hopes, desires, fears, and outrage. But I couldn’t answer, “What the heck is AI, anyway?”
While I understood Eric while we were talking, I found no retrievable home for this new knowledge anywhere in my neural network a month later during podcast production. Hear what I did there. I’m sounding more confident than I am, just like the voice of AI. Question and verify everything.
I will interrupt our recorded conversation from time to time to ask questions and report on answers I find from Eric or Claude. Claude isn’t another person in my network. Claude 3.5 Sonnet is an AI assistant computer program designed to interact with humans and help them through natural conversation. Think of it as software that can understand what you write and respond helpfully, like conversing with a knowledgeable person like Eric. Except Claude never sleeps and doesn’t play the harmonica.
What is AI?
Eric Pinaud: The question of the year or the past few years. Because, in part, these Gen AI tools are available to everybody. As opposed to in the past, technology was only accessible by programmers or techies or whatever else. Gen AI came about as a chatbot, and anybody who can write or listen can use it. And so they can use them better with some understanding of what it does well and not well. And so forth. So, we are going to talk about generative AI, and we’re going to start in the context of just a background of AI. In general, where have we been with AI? [Just a high-level overview of where we are today and what may be next. But some things are not here, and nobody knows exactly how they work, which is pretty crazy.
Many people may not consciously realize that AI has been around for a long time. It really started in the fifties, with mathematicians and computer scientists developing algorithms and so forth.
Health Hats: The first notable AI development in the 1950s was Arthur Samuel’s checkers program in 1952, which could learn and play the game independently. Another significant milestone was the Logic Theorist, developed in 1955 by Allen Newell and Herbert Simon, which could mimic human problem-solving skills.
Narrow AI – One prediction, one decision
Until about ten years ago, it was called the Era of Narrow AI.
Health Hats: 2010 to 2015.
Eric Pinaud: Yeah. Narrow AI refers to AI systems that can do something but are very narrow and specific. And you can only ask them to do something else if it’s language-based. It’s all code-based, and it’s AI. So, artificial intelligence is based on data analysis; AI makes predictions. All AI works like that to various degrees. You give it data. That’s the input. And the output is a prediction.
A basic example is the Netflix queue. The input is the stuff that I’ve watched. The output is a recommendation. However, intelligence in Netflix can only do that. It’s very narrow. At least until now, you can’t just talk to Netflix and say, Hey, I want to do this or that. They haven’t quite plugged that in yet. Maybe they will. I don’t know. But basically, the recommendation, Netflix, Spotify, and all those kinds of things are very narrow artificial intelligence. It does one thing based on these parameters that you give it. And then it gives you a prediction.
Broad AI – Natural Language
Eric Pinaud: Today, we’re in the age that they call Broad AI. So, things like Generative AI can do a little more. Generative AI, obviously, you can talk to it. It knows about a whole bunch of things, and you can ask it about pretty much anything, really. And you’ll get an answer. Sometimes, it’s a good answer, sometimes not so good.
AGI, Artificial General Intelligence – Creating Something New
Eric Pinaud: The future is what they call AGI, artificial general intelligence. And so, AGI is the holy grail, right? It essentially mimics the human brain’s ability to take a whole bunch of information from a whole bunch of different places and apply those connections to something new that you’ve never seen before. People can do that. Machines today cannot do that. Even Gen AI, even the latest, greatest models today, cannot do that. They cannot infer information that they know from other things to a new problem and apply that.
The holy grail is artificial general intelligence, which a lot of people are afraid of because, at that point, it might be better and faster than humans and could have some consequences that nobody really knows about.
Some people say that AGI might be here in the next five years. Some people say it’s going to take 30 years. Some people say we’re never going to get there. Nobody knows.
AI Examples
Eric Pinaud: We already talked about Narrow AI: Pandora and Netflix. Cruise control, I think, is a good one because everybody knows, oh, yeah. Many people know what cruise control is, and it used to be that cruise control; you would just press the button, and it would go at that speed. And at that point, it was not artificial intelligence because, at that point, all it did was lock a speed, and if the car in front of you slowed down and you didn’t brake, you’d crash right into it. Today’s adaptive cruise control has sensors in the car that take in a bunch of data from the car’s speed in front of you and then adapt. It predicts as to how fast or how slow you need to go. So, it’s broadened its scope. It is a much broader adaptation of that, but still artificial intelligence, not generative. It doesn’t create anything. Okay. Yeah. It just takes in some data, makes a prediction, and then applies it to the car going faster or slower.
Face ID
Health Hats: What about face ID? Is that narrow?
Eric Pinaud: Yes, face ID is narrow because it is a very narrow application of data points on a photo, and the answer is yes or no. That’s all it does. Even though it has improved, it can do so now if you have glasses on right. It’ll extrapolate a little bit and say, okay, yeah. That’s still you.
Health Hats: Whether I have my hat on or not or my glasses on, it’ll identify me.
Eric Pinaud: It’s getting there where it can. It has looser borders. Okay. And a slightly better understanding of what the variances might be.
Overwhelmed?
HEALTH HATS: I’m overwhelmed by how all the types of AI fit together, with their overlaps and subsets. You will hear many labels with AI, such as Narrow AI, Broad AI, Generative AI, and Artificial General Intelligence. Then there are types of AI: Machine Learning and Deep Learning, Natural Language Processing and Large Language Models, Predictive Analytics and Translation, Robotics, and speech and vision recognition. I suggest you just let this information and stories wash over you. Some will hook, but most won’t. That’s okay. It’s called learning by planting seeds. Hopefully, a drop of curiosity will feed a sprout. Onward.
ChatGPT
Eric Pinaud: OpenAI came out with ChatGPT two years ago, revolutionizing everything. Many companies have been working on Gen AI but haven’t released it for several reasons. But we could already see signs of generative AI in everyday things, like computer-type things. So, auto-completion in Microsoft Word is generative AI.
In your email, for example, you mentioned generative AI. And that’s been around for quite a while, but it hasn’t been very good. For me, at least, the things often suggest a few words, and I’m like, no, that’s not what I’m saying. Those models were very small, mostly local on your machine, with not much training data, and so forth, and that’s why the suggestions were not great. Of course, nowadays, we’ve got chatbots, ChatGPT from OpenAI, and a number of others, such as BARD from Google, Claude from Anthropic, and so forth.
Health Hats: Perplexity is one of those as well.
Eric Pinaud: Perplexity is a search engine that is plugged into a gen AI interface As opposed to ChatGPT
Perplexity has essentially combined a web search engine like Google with Gen AI. And so you can ask it questions in natural language. It answers you in natural language, but at the same time, it gives you the links To the various sources it found. There is a new thing called RAG, which is retrieval augmented generation. That is more code that people plug into something like Chat GPT so that if you ask it a question about something, it doesn’t know. It will then go in the background and get it right. There are very few models, and I don’t know which ones they are, but very few models have that built into them. But all these companies are experimenting with that as we speak.
Health Hats: One of the things I like is that I categorize things differently in my head. Not that’s the right way to do it. Is there a difference between using generative AI on anything like digital on the web? And then there’s where you’re; it’s like your information. You put in something you’ve written, and it’s asking something, or you’re putting in your medical record and asking what test you should have next. Are those different? Or is it the same tools that are just different data sources?
Hallucinations
Eric Pinaud: Same tool, different scope. That goes into what they call hallucinations. The broader the information in the gen AI system, the more likely it is that the answer that comes back to you will be either slightly wrong, slightly off, or completely made up, depending on your question. Because if you think about it, the data that they’re trained on is the internet. And for any topic, think about any topic. You can research it and find five people who say white and five who say black.
Health Hats: And that’s given equal weight?
Reinforcement Training
Eric Pinaud: Yeah. Pretty much. There are some nuances there. But at its core, yes. At its core, it takes all that stuff in. A part of the training for large language models is called reinforcement training, where they will ask questions, the system will come back with an answer, and then they’ll say thumbs up or thumbs down.
And that’s recorded and kept in memory. And you’re talking like thousands and millions of iterations of those things. So, the weight is no longer the same; in fact, that’s what these systems keep in memory. They’re weight-based algorithms that determine what is more likely to be the right word than another in any particular circumstance and string of words.
HEALTH HATS: Reinforcement training sounds like training a dog or child with treats or punishment. Or playing a video game and getting rewards for winning – getting to another level or messing up and getting killed. Trial and error. I worry about the tendency of people and institutions to maximize the reward without aligning ethics and values. In this podcast, we’ve talked about healthcare providers and consultants developing systems to increase survey and outcome scores without addressing the purpose of those results. Can AI do that, too?
I asked my grandson how he made decisions that weren’t simple. A simple decision could be taking a right or left. He has a great sense of direction. Instead, a decision about which course to take in school or which band to play in: he can struggle over decisions – so many factors and emotions and might make a list of pros and cons. Then, he realizes some factors or emotions are more important than others. Weighted differently – my words. Does AI do lists and weights? What goes into the weights? Can the factors include emotions? Who’s emotions? The programmer, the engineer, the internet source?
Stock Market Craziness
Eric Pinaud: Broad AI, we’ve got Gen AI and weather pattern predictions, which are AI-based. We know how those stock market predictions can go crazy, right? What was it a couple of years ago when it was just some video or game maker, and the stock market went crazy, so they had to stop it? Later on, general intelligence would probably invent viable new products. For example, today, you can ask Gen AI, and it’ll come up with a bunch of stuff that doesn’t make sense. The words together make sense. But the product itself wouldn’t make sense.
Would Gen AI know those things and only invent viable things? As I said, it makes inferences between various stuff it already knows about.
How is Generative AI different from Regular AI?
Generative AI creates things and generates new content. Regular AI creates nothing new, like your cruise control or the Pandora list. It gives you a prediction from the list of existing things. Gen AI, at its core, has a natural language input, some understanding, some reflection, and then generates an answer. And the answer is a string of words that all come one after the other. And the algorithms behind it tell it what the most likely next word is, which is fascinating. You can only do that if you have billions and billions of data points. That and billions of reinforcement learning and weights eventually give you the right word after the word that came before it and in context.
Health Hats: it’s funny that it, I don’t know, funny is not the right word, but, amazingly, it’s word by word.
Eric Pinaud: And it’s word by word but based on all the other words before it. Based on all the other texts that it’s seen before and understood, and so forth, an important thing to realize about Gen AI is that it’s core creative. AI, before that, never was creative. It was a computer program. It would give you the same thing every time you give it a particular input. It gives you the same output every time.
Gen AI is not like that. If you ask the same question three times in a row, you will get a different answer Three times in a row. And that is something that, as a computer programmer, my background as a computer programmer, if that had happened when I was writing code, it would’ve been a bad thing. It’s it needs to be reproducible. This is not fundamentally different from any computer science code that anybody’s ever written before. And the point of the whole thing. These models, basically there, have natural language processing at their core.
Deep Neural Networks
Eric Pinaud: And then they are being fed all kinds of information, essentially the entirety of the public internet. They ingest it in whatever manner that actually happens. And they remember it way better than we do. And then, when you ask a question. Again, natural language processing goes through what they call deep neural networks, right?
So, the brains of large language models are essentially modeled on human brains. They call them neurons and neural networks. They’re layers of pieces of code. When you visualize them in a diagram, they look like little dots and could be neurons or whatever else, and these things will fire in one way or another on each layer and go to the next thing and fire one way or another. And then come out with so some answer
Health Hats: Is that like people networking? I’m a networker, so I know thousands of people and always try to make connections. Is that like a manual Neural network, or I don’t I don’t know the neural part,
Eric Pinaud: You could think about it that way because it’s a network of a predetermined number of layers of millions of neurons each. And they connect in various ways. Those pathways get recorded as a good answer when the answer is correct. Reinforcement learning: I said this is on step one and that on step two, and this is on step three, and I was told it’s right. So, I’m going to remember that. Each of those knows about 17,000 possibilities or people they could talk to get further in the answer.
Learning in Harmony
Health Hats: My neurologist tells me that playing my horn is the best treatment for my Multiple Sclerosis. He says that music creates new neural pathways. My sax teacher tells me I can learn one thing at a time and constantly coaches me to slow down, which is my woodshedding (practicing). I clumsily figure out the fingering and breathing for a tricky measure or four, study the form or the chord structure, and slowly speed up. Learn one thing at a time – 10 minutes per, day after day. I’m like a baby learning to walk, talk, or eat – messy, funny, cute, trial and error, persistent, muscle memory-retaining patterns. I get a dopamine rush seeing my mind’s eye video short of the neural network spreading across my Swiss-cheese demyelinated brain. That rush motivates me to play almost every day. A little bit more, a little bit more.
Perhaps AI neural networks are similar, except they don’t sleep, have no other life, no 10-minute limit, or no dopamine rush. But they’re learning, with reinforcement of thousands/trillions of failures and successes.
Controllable, Explicable, and Predictable
I prompted Claude 3.0 Sonnet:
Generative AI is conceivable and actual, but we don’t know how it works. We can’t predict the output. Recommend three words to fill out the phrase: Gen AI is conceivable and actual, but not yet xxxx, where xxx could be something like understandable.
Claude’s output:
Let me think about three fitting words that could complete that phrase by considering different aspects of our current relationship with generative AI.
- “Controllable” – This captures how, despite being able to create AI systems, we still struggle to direct or constrain their outputs and behaviors with precision.
- “Explicable” – Similar to your suggestion of “understandable” but with a more technical connotation, highlighting our inability to explain the internal decision-making processes fully
- “Predictable” – This emphasizes our current limitation in reliably anticipating what these systems will generate, even when given the same inputs
Each of these words highlights a different aspect of the gap between our ability to create generative AI and our ability to grasp or control it fully.
Nobody Knows
Health Hats: The learning part is that if I want to talk about AI for the layperson, I know that Eric has some skills. So, I think about the, I retain, I retain patterns.
Eric Pinaud: Exactly. Yep. Okay. Yep. That’s exactly how the inside of these large language models works now. The thing that’s crazy to me is that nobody actually knows exactly how they work.
Health Hats: Even the programmer?
Eric Pinaud: The people who created large language models cannot tell you the answer when you ask the question. Okay? Nobody knows exactly. There are researchers now who are researching ChatGPT and other language models to try to figure out what the patterns are. Are they reproducible? Can we predict what is going to happen? As far as I know, nobody has broken through that, which is fascinating because all of the pieces of code are finite pieces of code that are very explainable, but there are millions of them with thousands of layers. And they can connect in different ways. Nobody knows how and why.
Prompt = Question
Health Hats: So, this word, prompt, is important.
Eric Pinaud: The prompt is your question.
Health Hats: Yeah. And that is, I know I think I’ve told you about my friend Amy Price, and what I’ve learned from her is she coaches us on how to ask questions. And how to go at it from different points of view. Or even, instead of wanting it to predict, you might be saying this wrong, using it to ask how to be clearer.
Modeled on the Brain
Eric Pinaud: Yeah, that’s a prompt. Again, a large language model is modeled on a brain. And when you think about talking to a large language model, it helps to think about talking to a person. Because when you speak to a person and ask a question, I. If you don’t give context, they’re going to be like, I’m not sure how about this. And some people might ask you, and they might say I’m not sure. Can you give me more information? But some people will make something up.
And that’s what Gen AI does. Okay. And so, the more context you give, the less it will make things up. Also, when you talk to a person, you ask them a question. They give you an answer. You might be like, are you sure? Are you sure about that? Because I’ve seen this thing, and it didn’t, it doesn’t quite align with what you’ve told me. And you can act and should act precisely the same way with gen AI.
Because it will essentially make up the answer a large portion of the time, they’re much better than they were just two years ago. Most of the time, they’ll be correct, but not always. And so, you can challenge and talk to it exactly like a person. Where did you get this info? Are you sure this is right? I’ve seen this over there; it’s a type of thing. Through those iterations, you refine the answer like you would talk with a real person. And get to something more compact and accurate.
Structured Data
So, one good thing to understand about AI versus gen AI is that artificial intelligence overall was only good at understanding structured data before generative AI and natural language understanding.
Health Hats: So that’s like fields with exactly acceptable responses?
Eric Pinaud: Exactly. It is well structured, like age. You can think of the things we work with regularly. What’s structured data, a spreadsheet? It is structured data. You have columns. Each column is specific, and you will find the same type of thing in each row. So, it might be a date, a number, or a piece of text. And so that is structured. You know what it is.
Health Hats: So, then the column is the, okay. It’s like the acceptable values, right? Like you, you won’t put happy in age?
Eric Pinaud: Exactly. If you have that and fed it to a normal artificial intelligence system, it would break it. It would cause an error or create something incomprehensible because you’ve just gotten bad data. So, spreadsheets were all those things that AI was very good at, right? Your cruise control and your car, all kinds of information coming back and forth from the sensor about the speed of the car in front of you, slowing down, going faster, your own speed, you know that, but it’s structured—the same type of data.
Unstructured Data
Eric Pinaud: Unstructured data is things like blog posts and podcasts.
Health Hats: A note that a doctor writes in the medical record instead of a checkbox.
Eric Pinaud: Exactly. A comment field, for example. There are places where you get both of those things in surveys. In surveys, you will have some fields where you can only pick from a dropdown, which will always have the same data. But then you have an open text box that will be unstructured. In the past, it was almost impossible to analyze surveys at scale in their entirety because of the unstructured data.
Generative AI understands language, and most of our unstructured data is language-based. Now, you can point generative AI to 5,000 survey responses that are a big text field and ask it to analyze and give you trends, summarize, and whatever else. It can do that. So that’s one of the fundamental differences between gen AI and regular artificial intelligence. Some of the other differences we’ve already talked about. Gen AI is creative, and because of that, it’s unpredictable. Computer systems in the past have always been very predictable, and that was essentially their nature.
Biases
Gen AI because it is based on the entirety of the internet and tends to be biased. It reflects our collective biases.
Health Hats: And the collective biases of the people who use the internet. Yes. If you’re talking about people who say somebody is unhoused and doesn’t have a cell phone, their experience isn’t there. Yeah. Or if somebody’s incarcerated, and I’m just saying it’s the people who, yeah, it’s interesting.
Eric Pinaud: Absolutely. And the other thing is the biases of the people who create the systems.
Health Hats: Say more about that.
Eric Pinaud: When you create those systems, you have to train them. At some point, you have to give them answers. You must put out guidelines. Those are people doing those tasks. So, for a long time, I didn’t know what the answer was today, but at the very beginning of image generators, generative AI for images, if you ask something like create an image of a doctor 90% of the time, what you would get would be an old white man.
Health Hats: I find that I do that like with clinicians. I have to say diverse, or I have to say a female doctor, or I have to say a young doctor and an old patient. It’ll produce a different image, depending on my guidance.
Eric Pinaud: Absolutely. And over the past couple of years, this stuff has evolved so fast. And so much so that the biases are still there. Companies have put more and more guidelines in place to reduce those biases, but they still exist. People create those systems. You know about some of the good things. One of the things I think is the easiest way for people to understand whether gen AI can be useful is if they work with content. I don’t work with content very much. I’ve only found Gen AI helpful in spots. Every so often, it’s useful because I need to generate a document or something, and I get an outline or something like that. But some people deal with content like you.
Time saver
Health Hats: I’m using text generation, question-and-answer text summarization, extraction, paraphrasing and rephrasing, image generation, classification, audio and video generation. I do any of that, and I find it amazing.
Eric Pinaud: The few times I’ve had a clear use for it, I’ve been blown away by how much time it saves.
Health Hats: And I find it, too. I’ve been using this for a few years, and it is much better. The change has been a logarithmic improvement. Amazing.
Scope, Context, and Bobbleheads
Eric Pinaud: I want to go back to something you asked earlier. I said it’s more accurate if the scope is smaller. When you use Chat GPT or some of these tools, you can have a question and give them context and a persona. You can be unbelievably detailed in either one of those two things. An example of a persona would be acting as a college professor. Do not speak condescendingly. Be very nice. Be aware that you have a PhD, and your undergraduate students do not. They just came out of high school. Therefore, explain things in detailed steps; that is all the additional information you can give. Use the system I’ve used to get your desired answer.
Health Hats: Yes. I do that. My non-techie thing is I have these bobbleheads by my desk, right? And they’re things like Rosie the Riveter and Scarecrow and anyway. And so, when I’m writing, I think this represents somebody who’s a solver. Or this is somebody who, you know, I, and so it’s, and now I’ve taken it. I’ve done that for years, but now, and I’ll say this is, then the audience is sleepy. And I’d like some humor. Give me five choices. Then, I put them together because there’s some humor in the summary, the title, or the content. It’s a little bit easier to digest. I’m just experimenting with that.
Experimenting
Eric Pinaud: We all are. Yeah. Even the people who proclaim to be experts are still experimenting. Nobody knows how the stuff works exactly. And now some people spend a lot of time and know quite a bit. But we’re all learning, and one of the cool things I think is that anybody can learn here because you don’t have, you don’t have to be a techie, you don’t have to write code, you don’t have to understand how it works. All you need to do is type in a question, have a conversation, keep iterating, and learn through that. You can tell the system who they are. You can tell the system who they’re talking to. Another piece you can do applies more to a very specialized system you might build. For example, a university career center built a chatbot based on Gen AI. They did the persona and the audience thing. Then, they did some testing on it. The students who tested it gave feedback that it’s okay, but it provides simple answers, and it doesn’t quite understand much of the context. What they ended up doing was—providing information as if you were onboarding a new staff member.
When you onboard a new staff member, you tell them a lot. Context, history, things that worked, things that didn’t work, why we’re here today, why it’s like this. All those kinds of things that you know usually are not written down. So they fed it, all that, and then they did more testing, and the students were blown away. They’re like, wow, they understand. And it gives me much more meaningful answers. And so forth. Oh, interesting.
That is not something everybody would do because you only do that when you’re doing something specialized. There are issues around copyright academic integrity, deepfakes, et cetera, and this could be the topic of three other conversations.
Sustainability
Eric Pinaud: These data centers use so much power and water. It’s obscene. It really is. I think last year it took something like $700,000 to run chat GPT for one day. The other piece of that is just the training part of the first version of Chat GPT that came out, so it came out two years ago. I think it was called Chat GPT-3 0.5, whatever it’s called. Just the training portion before they released it, right? They said it took the equivalent of electricity for 90 households for a whole year. Just to train that language model. And after that, the use every day was just like even more than that. Through the roof, right? So that’s not sustainable.
Part of the challenge will be finding better algorithms, different algorithms that don’t take so much computing power because we’re already hitting the limits. We’re hitting the limits of how much data we can train these models. They’ve already ingested the whole internet. Or the entire public internet. So now they’re trying to make deals with places that are not public and that are private for more data. But we’re hitting limits on how much power can be supplied to these things. Yesterday, we talked about the three-mile island nuclear reactor. Then, they want to reopen for the Microsoft AI data center. And maybe that happens, perhaps it doesn’t, but that shows you the scale of things.
Health Hats: It’s pretty crazy.
Eric Pinaud: People should be aware that they own your data whenever you upload anything to Chat GPT or any of those things. Whatever you gave them, whatever you uploaded to get your answers, they have it. They’re going to use it.
Networking to Solve a Problem
Health Hats: We were talking the other day, and I was telling you that one of the things I do in my work is go back to being a networker. What I try to do is host these mastermind groups. And what is the mastermind group that I have a topic for? Bringing people with diverse experiences together and chewing on a problem. So, how is that manual process a piece of this?
Eric Pinaud: By its nature, the large language models have that right because they have a wide range of information on a given topic, some of which might be wrong. But they do have a wide range of information. And in theory, we can synthesize that and give you various answers. I think we were talking the other day about making connections between different kinds of bodies of information, like being a generalist and knowing some about this topic, some about that topic, and some about this topic.
Health Hats: I know a little bit about a lot of healthcare. And the value of that.
Eric Pinaud: So, you can make connections between these things when faced with a new problem or question and come up with an answer or a potential answer based on making connections between those things.
Health Hats: So that’s like the neural network you talked about?
AGI Artificial General Intelligence – Approaching Human
Eric Pinaud: That is AGI, artificial general intelligence. We are not there yet. That is the holy grail. That is what all these companies want to try based on a problem you’ve never seen before.
Health Hats: Like what to do about climate, which we’ve not solved yet? Could it be either personal or societal?
Eric Pinaud: That’s right. Or neighborhood. The significant advantage machines would have is that they are much faster when they have the information. Eventually, if they had the ability to make these connections, they could have done it so much faster than we could. They don’t need to sleep. They don’t get tired.
People Solving a Problem, Asking a Question
Health Hats: But people still say this is what I would need an answer to. So, an example is I met these people from St. Louis, and for some reason, they found out that their pedestrian acts or fatality rate from accidents in their county was the highest in the country. And so they were wanting to solve that problem. They started with people just hanging out at the corner at those corners and recording stuff.
Once they started seeing patterns and figuring out, they worked with somebody who had developed a way to put a camera up and chew on the camera’s findings. These are improvements that might help this corner. And then they could move that from corner to corner. So, it started from a problem they cared about. For us, it might be the problem of caregiving, which is the challenge of staying healthy as a caregiver. So, it seems, in a way. It’s like for me, and being really into research with PCORI, who’s asking the questions? That’s the power of this for laypeople. They can ask the questions. Anybody can ask the question. That part of it is very democratic.
Any language
Eric Pinaud: It’s making things much more accessible. When have we ever had a powerful tool that’s … I was going to say accessible to everybody. That’s not true. You have to have access to a computer. If you don’t, go to a library and sit down. You need digital access. You also need to be able to formulate the question. It doesn’t have to be English. The systems understand all kinds of languages.
One of the things I did not too long ago was I had to write an email to a lawyer in France. Communication email or written communication in France is generally very formal, but when you’re speaking to a lawyer, it’s even way more. Even though I speak French fluently, I haven’t been to France for a long time, and I’ve never spoken directly with a lawyer. I went to Chat GPT and told them about it. I was like, Hey, I have to write this letter in French to a lawyer about these things. Here are the things I’d like the letter to sound like this, and it just spits it out in French perfectly. I changed a few things. Off I sent it.
Checking for Plagiarism
Health Hats: You know what I did this week? I finished writing the episode and had it run the plagiarism check feature to see if I had plagiarized. And it came up with Health Hats, which had said that before, and it pointed out the episode that I said it in. It said the second thing was something that I referenced. So, I already had a reference to it. And I thought, okay, that was an interesting experience. Because you want to give people credit. I’m the kind of person that anybody can use my stuff. I claim no intellectual property to anything I do, but that’s my choice, right? And I’m happy to use it because I’m giving and receiving. But not everybody’s like that. And I want to make sure that I credit people.
That’s what I want. I want people to credit me. They can use it, but I want credit. Yeah. And absolutely. How do you know that? And so that was the first time I tried that. Now I think I’m going to do that more often because then. I’ll put in that Eric said that, and Eric said that in 2019, in this particular place, I want to give Eric credit. I never thought about that before today.
Eric Pinaud: And that’s one of the things that people complain about as far as copyright violations for these language models are concerned; they are trained on much of the internet. And if you say, write a poem in the style of your favorite Robert Frost, it’ll just spit it out. But it won’t necessarily give credit. Like you, you could copy and paste that somewhere and pass it as your own.
Perplexity
Health Hats: One of the things I like about Perplexity is it shows you how it’s thinking, right? And then everything is referenced, right? And you can click it, and they’ll show you where they got stuff from. And then you can say, oh man, that’s just BS. They don’t know what they’re talking about. Or I didn’t realize they were experts in this. And then you can burrow in a little more.
Eric Pinaud: Yeah. I think some places for people who have to read, understand, and pay attention to a lot of text may be lawyers. I always hear about these bills that they write in Congress, and they’re 200 pages long, and people in Congress get them the night before the vote. The politicians don’t read them. Their aides try to read them. And then ask them questions, whatever. This would be a perfect thing. It feeds it to the engine and asks it the questions you want. And when the scope is so small that it’s one document, even if it’s 200 pages, that’s very small compared to the internet. The answers you will get will be very accurate. That’s a good use case.
Managing a Large Medical Record
Health Hats: / I know this guy who has two children who have rare diseases, and one of them is, seems to get a new rare disease diagnosis every year, every two years. And so his record is a mountain, right? And from all different sources, right? Because he sees 12 specialists or something. And so, he’s trying to use and feed the record. What are the patterns? And I will see a new cardiologist about X, Y, and Z. What questions should I ask? Yep. What tests should I bring? It makes a lot of sense.
Reflection
I’m getting more comfortable with this mental picture of AI’s similarity to blood and brain humans trying to have a relationship. The easiest to conjure up is labels. You and I would listen, ask questions, and provide answers. You ask AI Prompts, and AI offers Output. I’m unsure where listening comes in in this tech relationship analogy.
Meanwhile, I’ve learned that human behavior, language, and decision-making can be helpful models for understanding AI. Perhaps a deep understanding of AI is akin to understanding human emotions, culture, and motivation. When you think you know a bit, you realize there’s more to it. And when you think you understand a lot, your humility muscles get a workout. In any event, it’s fascinating. More to come.
Related episodes from Health Hats
Fear, Shame, Access, Connection -Privacy in Digital Exchange
Artificial Intelligence in Podcast Production
Health Hats, the Podcast, utilizes AI tools for production tasks such as editing, transcription, and content suggestions. While AI assists with various aspects, including image creation, most AI suggestions are modified. All creative decisions remain my own, with AI sources referenced as usual. Questions are welcome.
Creative Commons Licensing
This license enables reusers to distribute, remix, adapt, and build upon the material in any medium or format for noncommercial purposes only, and only so long as attribution is given to the creator. If you remix, adapt, or build upon the material, you must license the modified material under identical terms. CC BY-NC-SA includes the following elements:
BY: credit must be given to the creator. NC: Only noncommercial uses of the work are permitted.
SA: Adaptations must be shared under the same terms.
Please let me know. danny@health-hats.com. Material on this site created by others is theirs, and use follows their guidelines.
Disclaimer
The views and opinions presented in this podcast and publication are solely my responsibility and do not necessarily represent the views of the Patient-Centered Outcomes Research Institute® (PCORI®), its Board of Governors, or Methodology Committee. Danny van Leeuwen (Health Hats)